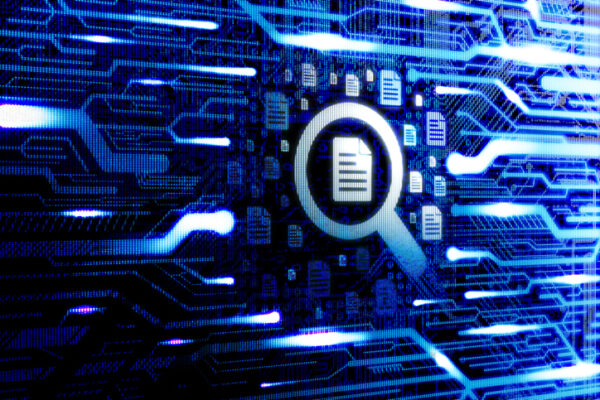
The accuracy and reliability of AI models hinges on the quality of the data they are trained on. This can’t be forgotten — especially when these tools are being applied to healthcare settings, where the stakes are high.
When developing or deploying new technologies, hospitals and healthcare AI developers must pay meticulous attention to the quality of training datasets, as well as take active steps to mitigate biases, said Divya Pathak, chief data officer at NYC Health + Hospitals, during a virtual panel held by Reuters Events last week.
“Garbage in is garbage out,” she declared.
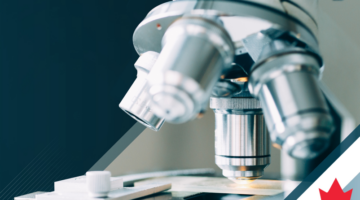
When Investment Rhymes with Canada
Canada has a proud history of achievement in the areas of science and technology, and the field of biomanufacturing and life sciences is no exception.
There are various forms of biases that can be present within data, Pathak noted.
For example, bias can emerge when certain demographics are over or underrepresented in a dataset, as this skews the model’s understanding of the broader population. Bias could also arise from historical inequalities or systemic discriminations present in the data. Additionally, there could be algorithmic biases. These reflect biases inherent in the algorithms themselves, which may disproportionately favor certain groups or outcomes due to the model’s design or training process.
One of the most important actions that hospitals and AI developers can take to mitigate these biases is to look at the population involved in the training data and make sure it matches the population on which the algorithm is being used, Pathak said.
For instance, her health system would not use an algorithm trained on patient data from people living in rural Nebraska. The demographics in a rural area versus New York City are too different for the model to perform reliably, she explained.
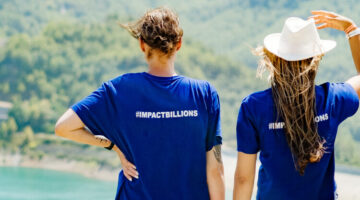
The Impact Brands: Empowering Wellness Through Natural and Holistic Solutions
In an era of escalating healthcare costs and a growing preference for natural, holistic approaches to health, The Impact Brands emerges as a collective of diverse brands dedicated to supporting overall wellness through natural means.
Pathak encouraged organizations developing healthcare AI models to create data validation teams who can identify bias before a dataset is used to train algorithms.
She also pointed out that bias isn’t just a problem that goes away after a quality training dataset has been established.
“Bias actually exists in the entirety of the AI lifecycle — all the way from ideation to deployment and evaluating outcomes. Having the right guardrails, frameworks and checklists at each stage of AI development is key to ensuring that we are able to remove as much bias as possible that propagates through that lifecycle,” Pathak remarked.
She added that she doesn’t believe bias can be removed altogether.
Humans are biased, and they are the ones who design algorithms as well as decide how to best put these models to use. Hospitals should be prepared to mitigate bias as much as possible — but shouldn’t have the expectation of a completely bias-free algorithm, Pathak explained.
Photo: Filograph, Getty Images