Over the past few years, a revolution has infiltrated the hallowed halls of healthcare — propelled not by novel surgical instruments or groundbreaking medications, but by lines of code and algorithms. Artificial intelligence has emerged as a power with such force that even as companies seek to leverage it to remake healthcare — be it in clinical workflows, back-office operations, administrative tasks, disease diagnosis or myriad other areas — there is a growing recognition that the technology needs to have guardrails.
Generative AI is advancing at an unprecedented pace, with rapid developments in algorithms enabling the creation of increasingly sophisticated and realistic content across various domains. This swift pace of innovation even inspired the issuance of a new executive order on October 30, which is meant to ensure the nation’s industries are developing and deploying novel AI models in a safe and trustworthy manner.
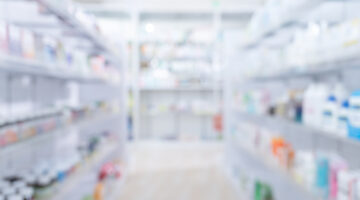
Unlocking Transparency in PBM Pricing
The TSX Venture Exchange has a strong history of helping early-stage health and life sciences companies raise patient capital for research and development.
For reasons that are obvious, the need for a robust framework governing AI deployment in healthcare has become more pressing than ever.
“The opportunity is extreme, but healthcare operates in a complex environment that is also very unforgiving to mistakes. So it is extremely challenging to introduce [AI] at an experimental level,” Xealth CEO Mike McSherry said in an interview.
McSherry’s startup works with health systems to help them integrate digital tools into providers’ workflows. He and many other leaders in the healthcare innovation field are grappling with tough questions about what responsible AI deployment looks like and which best practices providers should follow.
While these questions are complex and difficult to answers, leaders agree there are some concrete steps providers can take to ensure AI will be integrated more smoothly and equitably. And stakeholders within the industry seem to be getting more committed to collaborating on a shared set of best practices.
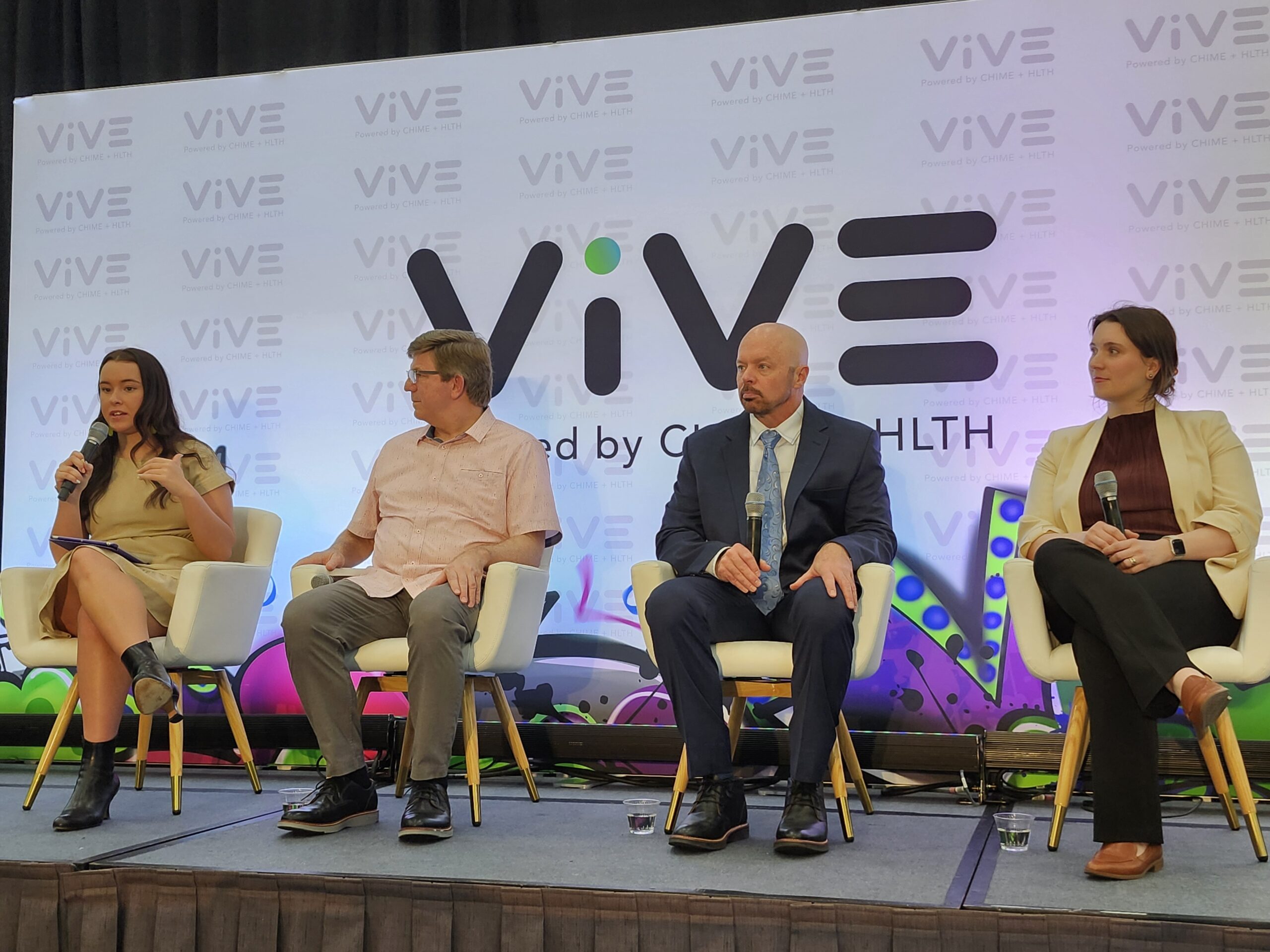
At ViVE 2024, Panelists Share Prior Authorization Progress and Frustration in Payer Insights Program
At the Payer Insights sessions on Day 1 of ViVE 2024, a panel on prior authorization offered compelling insights from speakers who shared the positive developments in this area after years of mounting frustration. Speakers also shared challenges as they work with providers to figure out how policy developments and technology will work in practice.
For instance, more than 30 health systems and payers from across the country came together last month to launch a collective called VALID AI — which stands for Vision, Alignment, Learning, Implementation and Dissemination of Validated Generative AI in Healthcare. The collective aims to explore use cases, risks and best practices for generative AI in healthcare and research, with hopes to accelerate responsible adoption of the technology across the sector.
Before providers begin deploying new AI models, there are some key questions they need ask. A few of the most important ones are detailed below.
What data was the AI trained on?
Making sure that AI models are trained on diverse datasets is one of the most important considerations providers should have. This ensures the model’s generalizability across a spectrum of patient demographics, health conditions and geographic regions. Data diversity also helps prevent biases and enhances the AI’s ability to deliver equitable and accurate insights for a wide range of individuals.
Without diverse datasets, there is a risk of developing AI systems that may inadvertently favor certain groups, which could cause disparities in diagnosis, treatment and overall patient outcomes, pointed out Ravi Thadhani, executive vice president of health affairs at Emory University.
“If the datasets are going to determine the algorithms that allow me to give care, they must represent the communities that I care for. Ethical issues are rampant because what often happens today is small datasets that are very specific are used to create algorithms that are then deployed on thousands of other people,” he explained.
The problem that Thadhani described is one of the factors that led to the failure of IBM Watson Health. The company’s AI was trained on data from Memorial Sloan Kettering — when the engine was applied to other healthcare settings, the patient populations differed significantly from MSK’s, prompting concern for performance issues.
To ensure they are in control of data quality, some providers use their own enterprise data when developing AI tools. But providers need to be wary that they are not inputting their organization’s data into publicly available generative models, such as ChatGPT, warned Ashish Atreja.
He is the chief information and digital health officer at UC Davis Health, as well as a key figure leading the VALID AI collective.
“If we just allow publicly available generative AI sets to utilize our enterprise-wide data and hospital data, then hospital data becomes under the cognitive intelligence of this publicly available AI set. So we have to put guardrails in place so that no sensitive, internal data is uploaded by hospital employees,” Atreja explained.
How are providers prioritizing value?
Healthcare has no shortage of inefficiencies, so there are hundreds of use cases for AI within the field, Atreja noted. With so many use cases to choose from, it can be quite difficult for providers to know which application to prioritize, he said.
“We are building and collecting measures for what we call the return-on-health framework,” Atreja declared. “We not only look at investment and value from hard dollars, but we also look at value that comes from enhancing patient experience, enhancing physician and clinician experience, enhancing patient safety and outcomes, as well as overall efficiency.”
This will help ensure that hospitals implement the most valuable AI tools in a timely manner, he explained.
Is AI deployment compliant when it comes to patient consent and cybersecurity?
One hugely valuable AI use case is ambient listening and documentation for patient visits, which seamlessly captures, transcribes and even organizes conversations during medical encounters. This technology reduces clinicians’ administrative burden while also fostering better communication and understanding between providers and patients, Atreja pointed out.
Ambient documentation tools, such as those made by Nuance and Abridge, are already exhibiting great potential to improve the healthcare experience for both clinicians and patients, but there are some important considerations that providers need to take before adopting these tools, Atreja said.
For example, providers need to let patients know that an AI tool is listening to them and obtain their consent, he explained. Providers must also ensure that the recording is used solely to help the clinician generate a note. This requires providers to have a deep understanding of the cybersecurity structure within the products they use — information from a patient encounter should not be at risk of leakage or transmitted to any third parties, Atreja remarked.
“We have to have legal and compliance measures in place to ensure the recording is ultimately shelved and only the transcript note is available. There is a high value in this use case, but we have to put the appropriate guardrails in place, not only from a consent perspective but also from a legal and compliance perspective,” he said.
Patient encounters with providers are not the only instance in which consent must be obtained. Chris Waugh, Sutter Health’s chief design and innovation officer, also said that providers need to obtain patient consent when using AI for whatever purpose. In his view, this boosts provider transparency and enhances patient trust.
“I think everyone deserves the right to know when AI has been empowered to do something that affects their care,” he declared.
Are clinical AI models keeping a human in the loop?
If AI is being used in a patient care setting, there needs to be a clinician sign-off, Waugh noted. For instance, some hospitals are using generative AI models to produce drafts that clinicians can use to respond to patients’ messages in the EHR. Additionally, some hospitals are using AI models to generate drafts of patient care plans post-discharge. These use cases alleviate clinician burnout by having them edit pieces of text rather than produce them entirely on their own.
It’s imperative that these types of messages are never sent out to patients without the approval of a clinician, Waugh explained.
McSherry, of Xealth, pointed out that having clinician sign-off doesn’t eliminate all risk, though.
If an AI tool requires clinician sign-off and typically produces accurate content, the clinician might fall into a rhythm where they are simply putting their rubber stamp on each piece of output without checking it closely, he said.
“It might be 99.9% accurate, but then that one time [the clinician] rubber stamps something that is erroneous, that could potentially lead to a negative ramification for the patient,” McSherry explained.
To prevent a situation like this, he thinks the providers should avoid using clinical tools that rely on AI to prescribe medications or diagnose conditions.
Are we ensuring that AI models perform well over time?
Whether a provider implements an AI model that was built in-house or sold to them by a vendor, the organization needs to make sure that the performance of this model is being benchmarked on a regular basis, said Alexandre Momeni, a partner at General Catalyst.
“We should be demanding that AI model builders give us comfort on a very continuous basis that their products are safe — not just at a single point in time, but at any given point in time,” he declared.
Healthcare environments are dynamic, with patient demographics, treatment protocols and diagnostic standards constantly evolving. Benchmarking an AI model at regular intervals allows providers to gauge its effectiveness over time, identifying potential drifts in performance that may arise due to shifts in patient populations or updates in medical guidelines.
Additionally, benchmarking serves as a risk mitigation strategy. By routinely assessing an AI model’s performance, providers can flag and address issues promptly, preventing potential patient care disruptions or compromised accuracy, Momeni explained.
In the rapidly advancing landscape of AI in healthcare, experts believe that vigilance in the evaluation and deployment of these technologies is not merely a best practice but an ethical imperative. As AI continues to evolve, providers must stay vigilant in assessing the value and performance of their models.
Photo: metamorworks, Getty Images