Each year, about 4.2 million people around the world die as a result of surgical complications that occurred within 30 days following a procedure. Not only do these surgical complications take many lives, but they also raise overall healthcare costs.
To address this problem, researchers at UPMC and the University of Pittsburgh created and deployed a machine learning algorithm that flags patients who are at high risk for post-surgical complications. On Friday, UPMC published research validating the tool in JAMA Network Open — the study found that the health system’s model outperforms the industry standard.
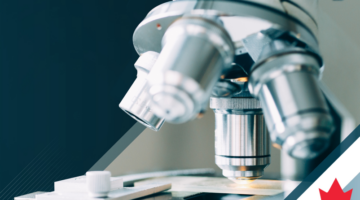
When Investment Rhymes with Canada
Canada has a proud history of achievement in the areas of science and technology, and the field of biomanufacturing and life sciences is no exception.
The research team trained the algorithm on medical records from more than 1.25 million UPMC patients. All of these patients had undergone a surgical procedure at one of UPMC’s hospitals from 2011 to 2019, said Dr. Aman Mahajan, the study’s lead author, in a recent interview.
The model was trained to identify patients who are at risk for mortality 30 days after their surgery, as well as flag patients who are at risk of a major cerebral or cardiac event, such as a stroke or heart attack.
A critical part of the algorithm’s design is that it was trained on patient data that “reflects the demographic profile of the state itself,” Dr. Mahajan pointed out.
“UPMC is a large health system. It’s got city tertiary care hospitals, it’s got community hospitals, it’s got some rural hospitals. So the population that’s represented here actually represents a fairly broad geographic and demographic profile,” he declared.
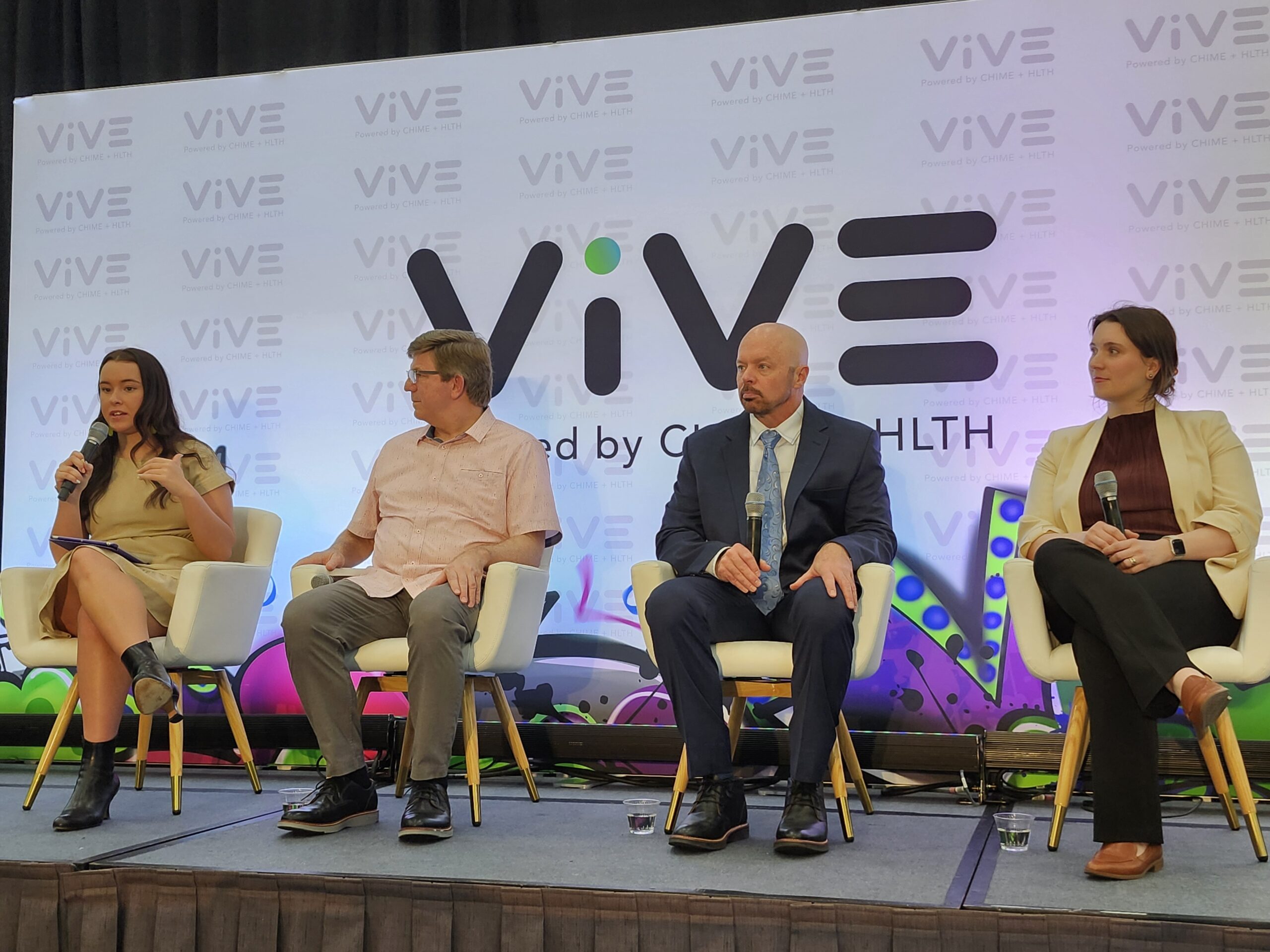
At ViVE 2024, Panelists Share Prior Authorization Progress and Frustration in Payer Insights Program
At the Payer Insights sessions on Day 1 of ViVE 2024, a panel on prior authorization offered compelling insights from speakers who shared the positive developments in this area after years of mounting frustration. Speakers also shared challenges as they work with providers to figure out how policy developments and technology will work in practice.
The AI model also considers patients’ social determinants of health, given these metrics often play a key role in determining how a patient will recover after an operation, Dr. Mahajan added.
To validate the model, UPMC deployed the algorithm and had it predict risk for more than 206,000 patients who were scheduled for surgery from June 1, 2019 through May 30, 2020. The health system then waited to see if the model’s predictions were right. For predictions of both mortality and cerebral/cardiac events, the algorithm was right more than 95% of the time.
The research team also compared their model against an industry standard, the American College of Surgeons’ National Surgical Quality Improvement Program (ACS NSQIP). Hospitals across the country use ACS NSQIP, but it requires busy clinicians to input patient data manually, and it cannot make a prediction if any information is missing. The study found that UPMC’s model did a better job at identifying high-risk patients than the ACS NSQIP.
Post-validation, UPMC deployed the algorithm across 20 of its hospitals. Each morning, the tool reads the electronic medical record for patients who are scheduled for surgery, and it flags those who are high-risk for complications. When clinical teams see this notification, they can intervene with precautionary measures, such as delaying the procedure or taking a patient off a certain medication. Clinicians can also run the model any time they wish, such as right before a patient visit, Dr. Mahajan added.
Consider a patient who is scheduled for a surgery to treat colon cancer. This patient’s risk profile could look very different depending on medical history and life circumstances — their comorbidities, medications, access to healthy food and previous medical events all play a role in determining how their recovery will play out.
“The surgeon’s office will tell you what the risk is and what your likelihood is for success — this successful surgery is in relation to all the other medical illnesses that you have. At that point in time, they can just run the risk score, and it takes them 30 seconds or less to actually get that. They can — at the point of first contact — be able to determine what might be the risk and that allows them to make better decisions,” Dr. Mahajan explained.
The model also brings patients into the process of shared decision-making, he pointed out. Patients can enter a dialogue with their providers about whether they think surgery is the best choice for them or if they should push the procedure back a few weeks to get other health problems under control.
Currently, UPMC’s model predicts patients’ risk of mortality and major cardiac and cerebral events. In the future, the health system is aiming to train the algorithm to predict the likelihood of sepsis, respiratory issues and other complications that frequently keep patients in the hospital after surgery.
Photo: Simonkr, Getty Images