Before a healthcare provider launches an AI pilot, it’s crucial that they determine which metrics they need to track. A lot of health systems don’t really do this, pointed out Bill Fera — principal and head of AI at Deloitte — during an interview last month.
By establishing the right metrics early on, the provider can quickly nix the pilot if the metrics show that the AI tool isn’t worth using, he explained. Many health systems don’t know which AI pilots to scale and which ones to stop because they aren’t tracking the right metrics — or aren’t tracking metrics at all — Fera remarked.
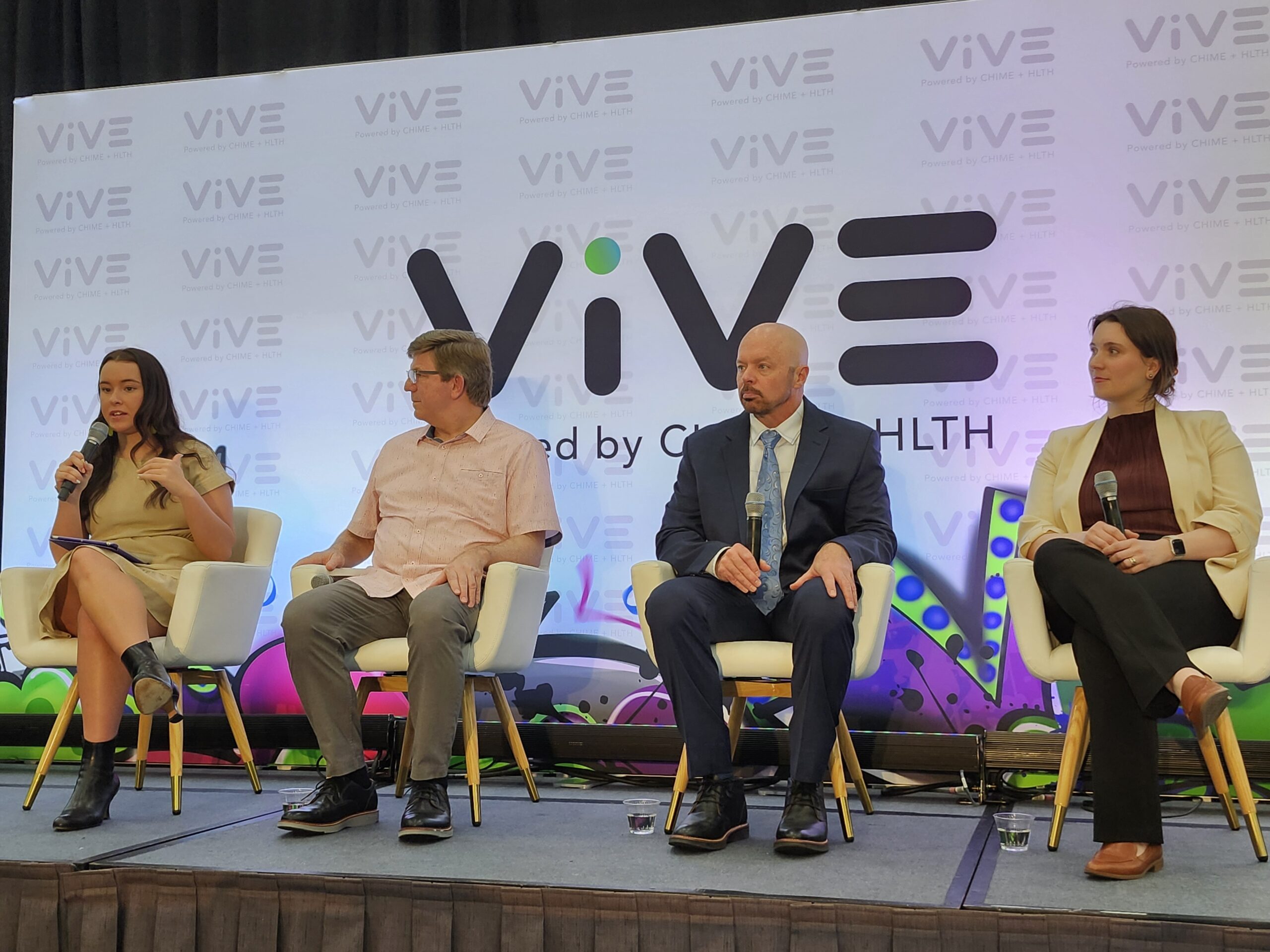
At ViVE 2024, Panelists Share Prior Authorization Progress and Frustration in Payer Insights Program
At the Payer Insights sessions on Day 1 of ViVE 2024, a panel on prior authorization offered compelling insights from speakers who shared the positive developments in this area after years of mounting frustration. Speakers also shared challenges as they work with providers to figure out how policy developments and technology will work in practice.
“There’s a lot of languishing in pilots that are inherently not going to create value. We’ve been really trying to work with our clients to prioritize use cases that can move the needle from a return perspective and establish the right metrics around that use case,” he declared.
In an interview this month during the HIMSS conference in Orlando, David Vawdrey — Geisinger’s chief data and informatics officer — agreed with Fera. He said health systems should spend more time designing their plan for evaluating success when it comes to tech pilots.
In Vawdrey’s view, the first question a health system must ask itself before deploying an AI tool is “What problem are we trying to solve?”
“If the problem is just ‘We want to deploy AI,’ then I guess it doesn’t matter what you deploy — you can write a press release and declare victory. But if you really want an impact and you care about the outcomes, you need to track the right metrics,” he stated.
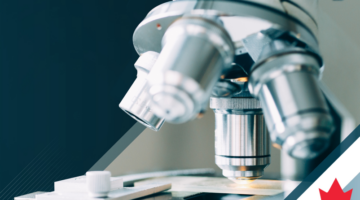
When Investment Rhymes with Canada
Canada has a proud history of achievement in the areas of science and technology, and the field of biomanufacturing and life sciences is no exception.
At Geisinger, the outcomes that matter most have to do with patient care and safety, Vawdrey noted.
So when it comes to the algorithms that Geisinger uses for things like cancer screenings or flu complications, the health system tracks these tools’ efficacy in terms of hospitalizations that have been prevented, lives that have been saved and spending that has been reduced, he said.
“Those are the things that we often don’t think about. Sometimes we, as an industry, throw technology in and hope to just sort it out later and assess whether it works. Oftentimes, that isn’t an effective strategy,” Vawdrey remarked. “We always try to have a rigorous evaluation plan before we ever deploy something.”
To form a strong evaluation plan, a health system must determine the problem it’s seeking to solve, which outcomes matter most, what success looks like, and the numbers they will look at to discover if the tool is working or not, he explained.
When the tool isn’t performing well, the health system must figure out if this was the result of a strategy problem or execution problem, Vawdrey added. If the problem had to do with the execution, there could very well be an opportunity to rework the pilot and try again, he pointed out.
Source: metamorworks, Getty Images