The first biotech revolution began 50 years ago when molecular biologists used DNA engineering to introduce a foreign genetic sequence into a bacteria and successfully produce a protein not encoded by the host genome. This revolutionary moment enabled a new era of scientific research that has radically advanced our understanding of how cells function in health and disease. It also opened the door to wholly new classes of therapies (recombinant proteins, monoclonal antibodies, targeted small molecules, gene and cell therapies, and gene editing) that have improved health outcomes for millions of patients.
Despite the transformative power of the first biotech revolution, traditional biopharmaceutical drug development paradigms continue to face significant R&D hurdles even after decades of advancement. There is a less than 10% attrition rate of therapies that make it to clinical trials and a roughly 9% success rate from Phase I to FDA approval, significant obstacles to translating molecular biology discoveries into the therapies needed to address the unmet medical needs of millions of people. These inefficiencies have resulted in billions of dollars wasted on failed R&D projects and patients being enrolled in clinical trials of investigational therapies from which they were unlikely to benefit. Obstacles persist even after product approval due to challenges in understanding how best to deploy novel therapies in real-world settings outside the highly defined patient populations evaluated in clinical trials.
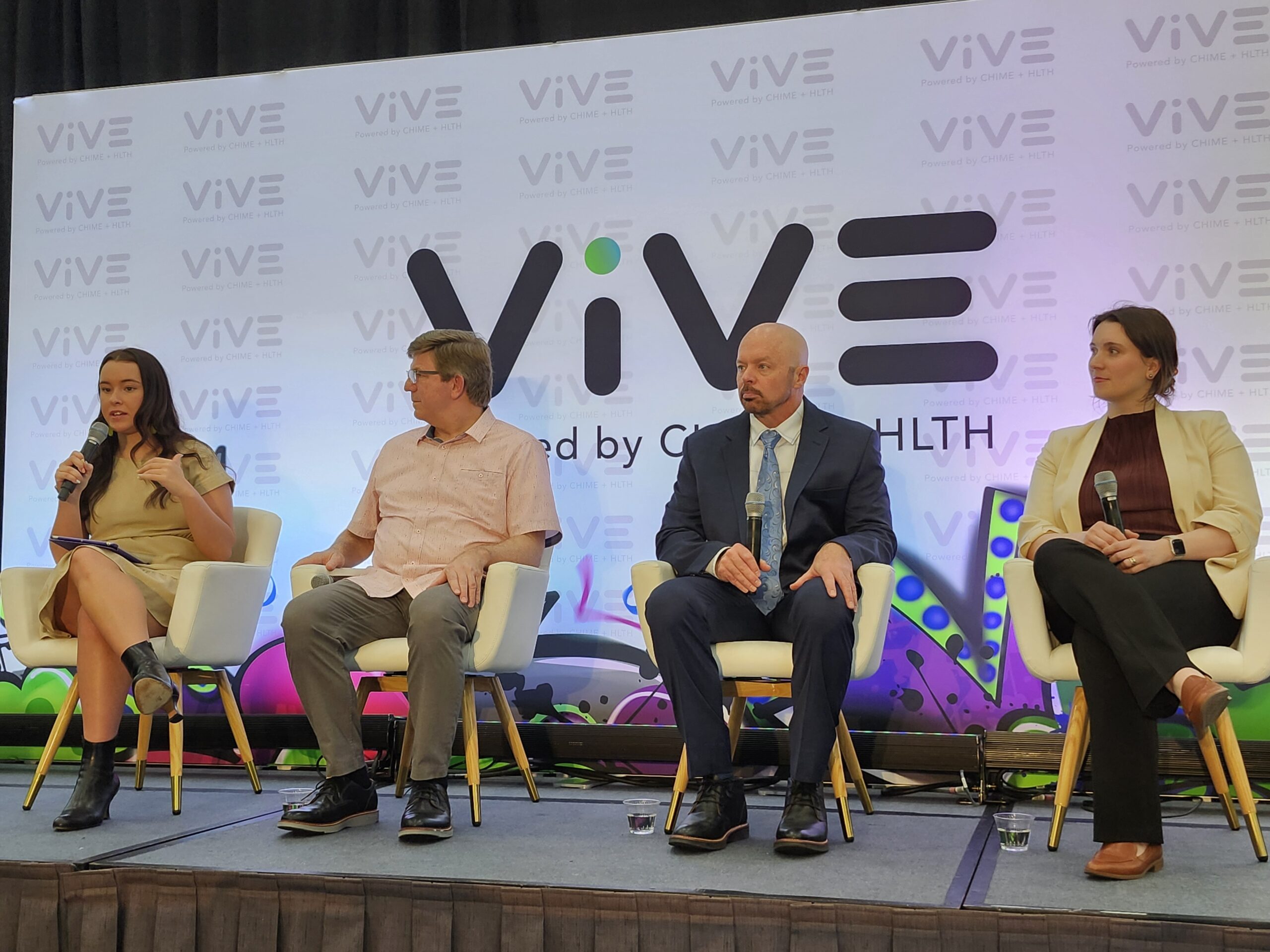
At ViVE 2024, Panelists Share Prior Authorization Progress and Frustration in Payer Insights Program
At the Payer Insights sessions on Day 1 of ViVE 2024, a panel on prior authorization offered compelling insights from speakers who shared the positive developments in this area after years of mounting frustration. Speakers also shared challenges as they work with providers to figure out how policy developments and technology will work in practice.
Getting beyond these bottlenecks requires a new approach to integrating biology and technology, led by advanced artificial intelligence (AI) and machine learning (ML) paradigms. Just as biologists used DNA engineering to catalyze the first biotech revolution, data scientists can engineer biology utilizing computation, enabling a new era of compute-enabled biotechnology companies. Technology-forward biotech — or tech-enabled bio — companies are driving tremendous advances in human health by structuring, analyzing, and extrapolating data from disparate sources to identify novel drug targets, design therapies optimized for safety and efficacy, enable novel diagnostic and prognostic tools, and identify patients most likely to benefit from a particular treatment. Equally important, these vast data sets have the power to radically reduce the time and cost of developing novel therapies and improve their use in real-world settings by allowing corporate and clinical decisions to be based on millions of real-world data points rather than predefined data inputs. This benefits patients, payers, and companies, and their investors.
Current discovery and development paradigms have multiple bottlenecks
Two critical limitations of traditional approaches to drug discovery and development are 1) the use of hypothesis-driven research and 2) the failure to leverage and incorporate data and insights regarding a particular drug target or therapeutic molecule that are scattered across the published literature and multiple data sources. These limitations narrow the scope of discovery and development to areas already known to be relevant to a particular biological pathway or disease indication, resulting in less than fully informed decision-making. They also are key reasons that bringing a new drug market on average takes more than ten years and $1 billion. Tech-enabled bio companies offer a new path around these bottlenecks by developing closed-loop AI- and ML-based platforms that can accelerate the design-build-test-learn (DBTL) cycle in life sciences. These compute-enabled platforms can extrapolate heterogeneous data to reduce the amount of time, experimentation, and costs associated with drug hit, target, and lead generation, as well as clinical trial design, patient stratification, and enrollment. These tech-enabled companies have used AI/ML to significantly reduce the preclinical R&D timeline, in which companies can now go from a hit to a viable lead candidate drug in less than 18 months and less than a million dollars compared to several years and tens of millions spent.
The tech-enabled bio revolution is here
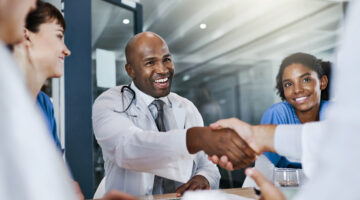
Using Informed Awareness to Transform Care Coordination and Improve the Clinical and Patient Experience
This eBook, in collaboration with Care Logistics, details how hospitals and health systems can facilitate more effective decision-making by operationalizing elevated awareness.
Generative AI technologies, such as those used in ChatGPT, are supercharging the tech-enabled biology revolution by enabling de novo discovery and development of entirely new drugs from scratch. This is feasible because, unlike hypothesis-driven approaches in which research is based on something already known, the insights gained by analyzing millions of existing data points without the constraints of predefined data inputs or output rules are entirely novel. Furthermore, these companies can create “digital twins” of animal and patient models utilizing AI, in which these robust multi-model biosimulations could open the door to completely digitized therapeutic asset development. Generative AI is already being deployed to enable “multi-omics” target discovery (i.e., identifying factors that contribute to disease through interaction with other proteins or pathways that may not appear relevant when analyzed individually). The use of deep biology analyses can considerably reduce the time needed to discover and prioritize novel targets from several months to just a few clicks of the mouse. This same approach can be applied to generating novel therapeutic molecules through the use of automated, ML-based drug design processes that can identify lead-like molecules in a week rather than months or years. AI and ML technologies are also being used to design and predict outcomes for clinical trials by analyzing real-world patient data to identify trial participants most likely to benefit from the therapy being tested. Insights gained from these technologies can radically reduce the size, cost, failure risk, and duration of clinical trials. Tech-enabled bio companies are utilizing computation for patient stratification to create a new era of precision medicine whereby patient outcomes are dramatically improved by systematically identifying the best treatment/therapeutic intervention for an individual based on their unique phenotypic and genotypic expression profile. Large troves of EHR data can now be tagged, labeled, and structured at scale to enable predictive analytics, genomic data analysis, phenotypic stratification, and treatment optimization. We can now begin to predict how specific subgroups of patients will respond to a given treatment protocol and how treatment regimens can be optimized for maximum therapeutic benefit.
The benefits of digitalizing life science R&D workflows, including wet lab experiments, high-throughput compound screening, animal models, and extensive clinical trials, cannot be overstated. These fragmented workflows contribute significantly to the time, cost, and risk bottlenecks that have long plagued traditional drug development and treatment strategies. The new era of full-stack compute-enabled bio companies automating, optimizing, and connecting these siloed workflows and enabling the transformation of previously disparate data into actionable insights will drive incredible advances in human health. The next industrial revolution is here.
Photo: Alfred Pasieka/Science Photo Library, Getty Images, http://www.gettyimages.com/license/680792467
Krish Ramadurai is a Partner at Harmonix Fund where he leads the firm’s technical due diligence, investment sourcing, and portfolio operational support across their healthcare, life sciences, and deep technology practices. He is a multi-published author, advisor, lecturer, and former scientific researcher at Harvard and MIT. While at Harvard, he worked with Nobel Laureate Economist Michael Kremer on the United States Agency for International Development’s Innovation Ventures Fund and authored three books on medicine and applied engineering. Krish serves as a venture advisor for Nucleate (a global bioentrepreneur community) and is a board observer for Sorcero and xCures.
Notably, he has received nominations for esteemed industry accolades, including Forbes 30 Under 30 in venture capital and the NVCA Rising Star Award.
Krish has advanced degrees in biology, nanotechnology and nanomedicine, and business administration from Harvard University, Oxford University, and Washington University in St. Louis, respectively, and a bachelor’s degree in integrative biology and economics with a chemistry minor from the University of Illinois at Urbana-Champaign. In his spare time, he enjoys traveling and is an avid classic car enthusiast.
This post appears through the MedCity Influencers program. Anyone can publish their perspective on business and innovation in healthcare on MedCity News through MedCity Influencers. Click here to find out how.