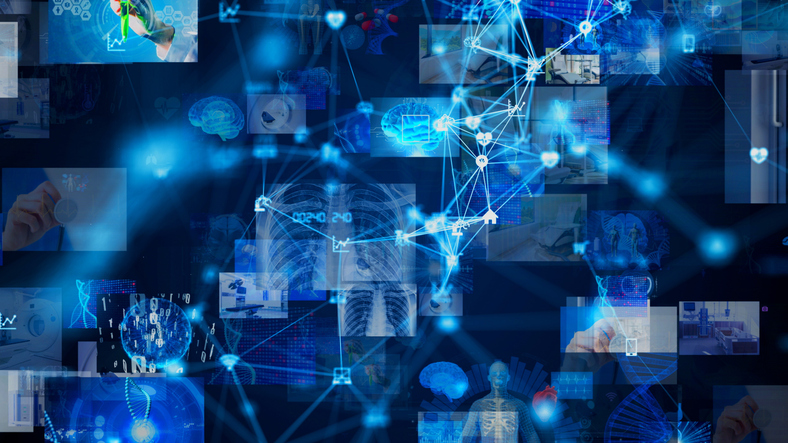
This article is part of a series on the use of algorithms in healthcare and is part of a Fellowship supported by USC Annenberg Center for Health Journalism. Read the first part of the series, on risky algorithms used by hospitals during the pandemic, or listen to our podcast episode about it.
Similar to a lot of digital technologies, artificial intelligence tools in healthcare have been buoyed by the pandemic. The Food and Drug Administration propelled a record number of devices to the market last year, including an emergency use authorization for a tool to predict which patients were more likely to experience complications from Covid-19. It also cleared a tool to flag potential cases of pulmonary embolism in CT scans.
At the same time, more hospitals are starting efforts to build their own algorithms using patient data. Mayo Clinic recently spun out a startup to commercialize algorithms for the early detection of some types of heart disease, and Google struck a partnership with HCA to access de-identified records from its 184 hospitals to develop healthcare algorithms.
As AI tools have gone from nonclinical to clinical applications, FDA has felt the need to regulate their use in healthcare. But regulating these emerging tools is a tall order. Back in 2019, the FDA started taking a closer look at how to address questions about the safety and accuracy of these algorithms over time. But in the years that have passed since, the influx of AI-based software tools has outpaced the agency’s efforts to build thoughtful regulations.
A review published in Nature of 130 FDA-cleared AI devices over the last five years found the majority had only been tested retrospectively, which makes it difficult to know how well they operate in a clinical setting. In fact, more than half of them had been cleared just last year.
The FDA acknowledged the surge in companies approaching the agency with AI- or machine learning-based software.
“Because of the rapid pace of innovation in the AI/ML medical device space, and the dramatic increase in AI/ML-related submissions to the Agency, we have been working to develop a regulatory framework tailored to these technologies and that would provide a more scalable approach to their oversight,” FDA spokesperson Jeremy Kahn wrote in an email to MedCity News.
As the FDA drafts up new regulations to evaluate these tools, several big questions loom: How will regulators handle questions about bias and fairness? And what information will patients and their doctors have about these systems?
Getting this right is important. Where a decision by a doctor might only affect a few patients, these algorithms could touch thousands of lives.
Put another way: “The risks can be so great — death — but so can the benefits — life,” said Patrick Lin, director of the Ethics and Emerging Sciences Group at California Polytechnic University- San Luis Obispo. “That makes healthcare one of the trickiest areas of technology ethics. The risk-benefit calculation can be heavily skewed, given how big the stakes are.”
More guidance planned for this year
Several types of algorithms currently aren’t reviewed by the FDA at all, such as symptom checker “chatbots” and certain types of software tools that suggest the best course of action for clinicians. The agency plans to expand oversight of these types of software according to a draft guidance that’s expected to be finalized this year, especially for algorithms used to treat serious conditions or where the user can’t review the basis for the suggestion.
Some groups, including the American Hospital Association, pushed back against the initial guidance two years ago, warning that the changes “could result in many existing (clinical decision support) algorithms being subject to the FDA approval process and ultimately slow the pace of innovation.”
Meanwhile, the FDA’s new Digital Health Center of Excellence, which was created last year, has been staffing up to establish best practices and more closely evaluate how software tools are regulated in healthcare.
An action plan shared by the FDA at the beginning of the year hinted how the agency is thinking about regulating these devices in the future, though details were sparse.
“There are details that need to be fleshed out, but I think they hit on a lot of the same issues that people had been thinking about,” said Christina Silcox, a digital health policy fellow at the Duke-Margolis Center for Health Policy.
For example, the agency is looking to build a framework to approve future changes in algorithms. While developers often tout machine learning tools’ ability to adapt or improve over time, for the most part, the FDA has only cleared algorithms that are fixed or “locked.”
Developers would have to share what exactly they plan to change, and how it would be safe, as part of the clearance process. The FDA also plans to release more details in a draft guidance later this year.
This is important because algorithms can become less accurate over time, as clinical practices change, new treatments are introduced or the data itself changes. Additionally, updates could be used to make a model more accurate for a specific location where it is implemented.
“One of the concerns is as you move algorithms to different places and they have completely different populations, you may need to tune those algorithms to work for those populations,” Silcox said. “Having a way of building into (clearance) how to do that is a really creative idea on the FDA’s part.”
A ‘nutrition label’ for AI
Given that most AI systems are written off as proprietary, it can be difficult to know how — and how well — they work. The FDA also mentioned plans for making software tools more transparent and ensuring they are unbiased, though it hasn’t yet shared how it plans to achieve this.
One idea is to create a “nutrition label” of sorts so that a system’s capabilities and limitations are clear to the clinicians that would be using them, Silcox said. For example, it would be important to know if a model had been tested prospectively, or if it works well in patients of different races, genders, ages, with different comorbidities or in different locations.
“There could be more transparency with the labeling — being able to know in the labeling, what populations has this algorithm been trained on and tested on, and how was the testing done,” she said.
Just as patients know what Covid-19 vaccine they’re getting, who developed it, and its efficacy, patients would also want to know about an AI’s qualifications, Cal Poly’s Lin said.
“You might want to give patients a choice, the opportunity to opt out or switch to another model,” he said.
In a recent survey of health systems conducted by MedCity News, 12 said they were using clinical decision support tools related to Covid-19. Most of them said the software they used had some indicator so clinicians could know what risk factors went into a score, but when it came to patient transparency, almost none of the hospitals specifically told patients that an algorithm was involved in their care.
Telling a patient that they have a risk score of 20 — or another number — would probably not be very helpful, but telling a patient that they’re in the top 5% for risk could grab their attention, Silcox said. People should also be aware if software is helping make an important decision, such a device cleared by the FDA to screen for potential cases of diabetic retinopathy using an image of patients’ eyes.
“One thing you want to make sure of is are you asking more of the software than any other test?” she said. “Someone knows if they’re getting labs done. Some sort of blood got drawn or some sort of sample got taken. A lot of the software can be really invisible to a patient and it is good for them to know that a critical piece of information came from the AI.”
This also brings up some bigger, knottier ethical questions that aren’t really addressed by regulation. For example, how do clinicians actually interact with these types of software, and in the long term, will it change the way they practice?
“We tend to overtrust systems that seem to work decently well. You can imagine if an AI system or an app spat out a diagnostic, there’s a serious risk of scenario fulfillment,” Lin said. “At most, the human has a veto power, but they’re going to be reluctant to use it.”
The level of regulatory scrutiny brought by the FDA, and whether a system has gotten clearance, may ultimately be a deciding factor in whether clinicians use these tools at all.
Elise Reuter reported this story while participating in the USC Annenberg Center for Health Journalism’s 2020 Data Fellowship.
The Center for Health Journalism supports journalists as they investigate health challenges and solutions in their communities. Its online community hosts an interdisciplinary conversation about community health, social determinants, child and family well-being and journalism.
Photo credit: metamorworks, Getty Images